This competitive corporate environment makes maintaining great people more important than ever. High staff turnover disrupts business and costs a lot to attract and train new hires. Recent data analytics advances allow firms to better forecast and reduce retention risk. This blog article discusses how firms may utilize analytics to detect staff turnover issues and establish retention strategies.
Table of Contents
- The cost of losing talent
- What is retention risk?
- Role of analytics in identifying retention risk
- Data collection and analysis in employee retention risk
- Strategies for mitigating retention risk using analytics
- Challenges and considerations in using analytics for employee retention
- Future trends in retention analytics
The cost of losing talent
Understand staff turnover’s impact before going into data. Employee turnover lowers productivity since they leave with knowledge and skills. Finding the appropriate replacement can be expensive and time-consuming, requiring months. New hires’ integration into business culture and workflows can be messy, causing more inefficiencies and errors.
What is retention risk?
Employee retention risk is the possibility of leaving an organization within a specific timeframe. This risk is affected by job satisfaction, management, compensation, career advancement, and work-life balance. Identifying these elements can aid retention methods.
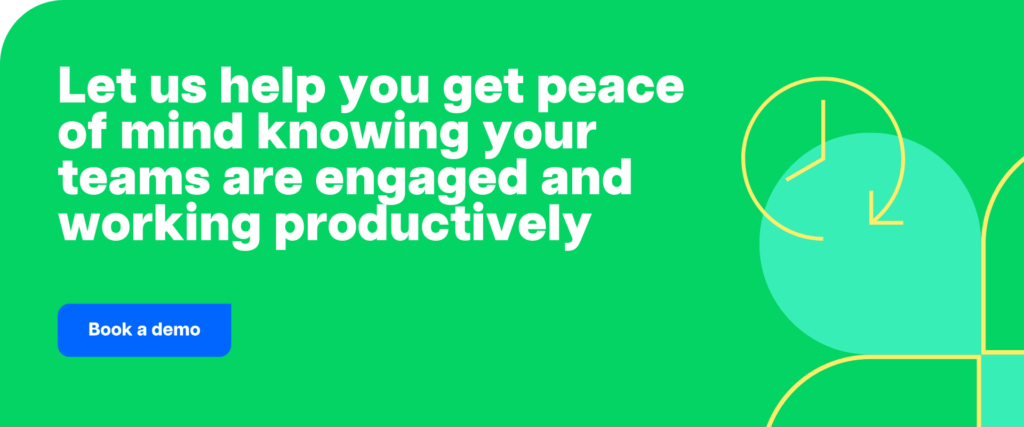
Role of analytics in identifying retention risk
Analytics in HR has transformed employee retention. HR professionals can identify retention issues and execute solutions using various analytical tools. This part discusses HR analytics and how predictive analytics may predict turnover for proactive retention measures.
Overview of analytical methods and tools used in HR
HR analytics extends from descriptive analytics that summarize previous occurrences to predictive and prescriptive analytics that predict future events and propose actions. Here are some popular analytical tools and methods:
- Descriptive analytics: Involves analyzing historical data to understand trends and patterns. Tools like HR dashboards and reports help visualize aspects such as turnover rates, employee satisfaction scores, and other key HR metrics.
- Predictive analytics: This uses statistical models and machine learning algorithms to predict future occurrences based on historical data. Tools such as IBM Watson, SAP SuccessFactors, and others are popular for their predictive capabilities, enabling HR to foresee potential turnover and understand the underlying factors.
- Prescriptive analytics: This type of analytics goes a step further. It not only predicts outcomes but also suggests actions to achieve desired results. It involves complex algorithms and is often integrated into advanced HR management systems.
- Sentiment analysis: Applied to employee feedback and surveys, sentiment analysis helps gauge the mood and morale within the organization, which can indicate potential retention issues.
- Network analysis: This looks at the relationships and influence patterns among employees to identify central figures whose potential departure might cause significant disruption.
Predictive analytics and forecasting turnover
HR predictive analytics analyzes employee data to predict turnover. Examples of these data points include job satisfaction, performance ratings, engagement scores, wage increases, promotion histories, and industry employment trends.
Predictive algorithms can tell HR if a department’s employees are at high turnover risk owing to managerial style or workload difficulties. The organization can intervene early by changing workloads, addressing managerial behaviors, or providing more assistance and appreciation to affected personnel.
These analytical tools and methodologies help HR professionals turn data into meaningful insights and improve employee retention. This maintains top talent and boosts organizational efficiency and employee happiness.
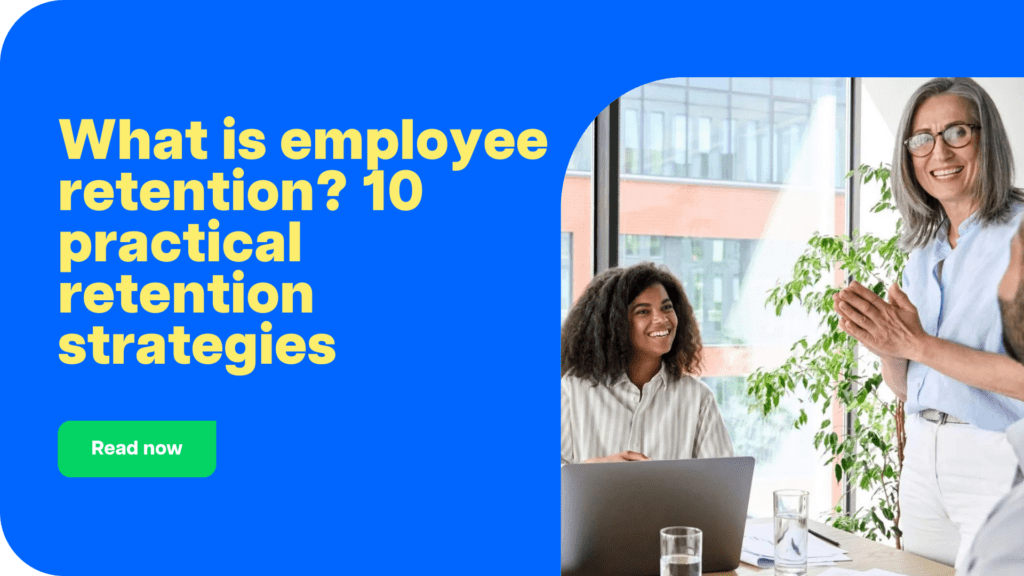
Data collection and analysis in employee retention risk
Accurate data collection and analysis are key to employee retention management. This data helps identify retention risk factors and develop effective solutions. Below, we discuss the types of data needed to analyze retention risk, how to gather and ensure quality data, and how to analyze this data to gain insights.
Types of data needed to analyze retention risk
HR professionals need data from several sources to evaluate and anticipate retention risks:
- Employee surveys: Gathering job satisfaction, engagement, work environment, and organizational culture feedback. Regular surveys should track changes and trends.
- Performance metrics: Performance metrics include personnel productivity, quality, and goal achievement. They can help determine whether poor performance leads to turnover.
- Exit interviews: Employees’ reasons for leaving. This data is crucial since it shows what’s improving or worsening in the organization.
- Attendance records: Absenteeism patterns can indicate disengagement or unhappiness.
- Demographic data: Age, tenure, department, role, and salary. This helps determine if specific groups have higher turnover.
- Engagement scores: Employee engagement assessments measure excitement, drive, and organizational commitment.
Techniques for effective data collection and ensuring data quality
For accurate and reliable analysis, high-quality data must be collected. Here are some methods:
- Ensuring anonymity and confidentiality: This encourages honesty in employee responses, particularly in surveys and exit interviews.
- Regular data collection: Regularly updating data collection processes to capture the most relevant information as the organization evolves.
- Multi-source data collection: Gathering data from multiple sources to validate findings and reduce biases. For example, combining survey data with performance metrics and exit interview insights.
- Standardization of data collection methods: Using standardized methods across the organization ensures that data is comparable and consistent.
- Training for data collectors: Ensuring that individuals involved in data collection are trained to do so effectively and ethically.
Methodologies for analyzing data to identify patterns and risk factors
After collecting data, numerous analytical methods can identify employee turnover patterns and risk factors:
- Regression analysis: Estimates variable relationships. It can identify which factors—salary, job level, or management relationship—affect employee retention.
- Machine learning: Historical data can teach algorithms to forecast staff turnover. Decision trees, random forests, and neural networks can discover complicated data patterns and relationships that cause employee turnover.
- Time series analysis: Useful for spotting trends over time, especially when examining retention strategies or attrition rates after organizational changes.
- Cluster analysis: These methods cluster personnel by similarity across variables. It can detect high-turnover groups.
- Survival analysis: This method measures the time until one or more events, like as turnover, occur and can help determine the influence of corporate policies or changes.
These data collecting and analysis methods help firms identify and address employee turnover causes. This proactive strategy boosts organizational performance, morale, and talent retention.
Strategies for mitigating retention risk using analytics
Using analytics to reduce retention concerns requires turning raw data into usable insights. These findings can help adjust retention strategies to employee requirements and business situations. Here’s how firms may use analytical insights, personalize retention strategy, and grasp leadership’s role.
Developing targeted retention strategies using analytical insights
- Identifying high-risk groups: Using predictive analytics, determine which employee categories are more likely to leave. This can depend on employment role, department, tenure, or demographics.
- Understanding underlying causes: Dig into the facts to find out why employees are unhappy. Do they leave for lack of advancement, remuneration, or work-life balance? Customizing tactics to address these difficulties can boost retention.
- Segmenting the workforce: Employees have various requirements and motives. Analytics can help segment the workforce by qualities and create retention programs for each segment.
- Benchmarking against industry standards: Compare retention data to industry standards to assess the organization’s performance and areas for development. This helps develop achievable goals and tactics.
Implementation of personalized retention plans based on analytics
- Personalized development plans: Create staff development plans based on survey performance and career goals. Training, mentorship, and professional paths are examples.
- Flexible working conditions: Analytics can show if specific groups prefer flexible working arrangements. Remote work, flexible hours, and a four-day workweek may help retain individuals who prioritize work-life balance.
- Reward and recognition programs: Use data-driven rewards to acknowledge exceptional performance, loyalty, innovation, and teamwork. Rewards tailored to employee values boost satisfaction and loyalty.
- Health and well-being initiatives: If stress or burnout rates are high, organizations may explore wellness programs, mental health days, or health benefits.
Role of leadership and management in executing data-driven retention strategies
- Championing the use of analytics: Leaders must promote analytics in HR decision-making. Analytics tools and HR training are included.
- Communication and transparency: Leaders should explain how data improves the workplace. Employee trust and buy-in can rise with decision transparency.
- Implementing changes based on insights: Leaders must not just collect data but also act on the insights provided. This might involve changing management practices, adjusting policies, or reshaping corporate culture.
- Monitoring and adjusting strategies: Leadership should carefully monitor strategy efficacy and adapt to new data and changing situations.
Analytics in retention requires a data-driven culture. Leadership and management can retain key employees and boost health and productivity with analytics insights. This proactive retention strategy addresses issues before they generate turnover.
Challenges and considerations in using analytics for employee retention
When utilizing analytics to address employee retention risks, organizations must navigate several challenges and considerations to ensure effectiveness and maintain trust.
Addressing ethical concerns related to employee data and analytics
- Privacy and consent: Organizations must handle employee data carefully, ensuring that personal information is collected and used with consent and kept confidential.
- Bias and fairness: There is a risk that analytics models may perpetuate existing biases if not carefully designed. Ensuring that the data and algorithms used are fair and unbiased is crucial.
- Transparency: Employees should be informed about what data is being collected and how it will be used. This transparency helps build trust and reinforces the ethical use of data.
Limitations of relying solely on data for retention-related decisions
- Incomplete data: Data might not capture all dimensions of employee experience, such as interpersonal relationships and external life events, which can significantly influence retention.
- Changing dynamics: What works today may not be effective tomorrow. Relying solely on historical data without considering changing workforce dynamics can lead to outdated or ineffective decisions.
- Overdependence on technology: Excessive reliance on analytics can lead to neglecting the human aspects of HR, such as personal interactions and leadership intuition, which are vital for understanding and influencing employee motivations and behaviors.
The importance of integrating human judgment with analytical insights
- Contextual interpretation: Human judgment is essential to interpret data within the proper context, understanding nuances that the data alone might not reveal.
- Ethical decision-making: Humans can consider ethical implications in ways that algorithms cannot, ensuring that decisions are fair and just.
- Adaptive strategies: Human insights can help adapt strategies dynamically, responding to new trends and individual cases that the analytics might not yet recognize or have been programmed to handle.
Organizations can balance analytics with human resource management by addressing these ethical problems, acknowledging data limitations, and valuing human judgment. This comprehensive approach makes retention tactics data-informed and human-centered, improving effectiveness and sustainability.
Future trends in retention analytics
Retention analytics tools and methods evolve with technology. Organizations may improve staff retention with AI, machine learning, and data integration platforms.
- Advanced predictive analytics: Integrating external market data and real-time sentiment research into predictive models will improve employee turnover projections.
- AI and automation: HR managers may focus on strategy and employee engagement by automating analytical activities with AI. These solutions also notify users about retention issues in real time.
- Integrated data systems: Integrating HR systems like performance management, employee engagement, and learning can give a more complete picture of employee experiences and retention drivers.
- Personalized employee experiences: Analytics will allow more targeted staff management, from onboarding to professional growth, to match individual preferences and career goals.
Conclusion
As we’ve discussed, analytics is changing employee retention. Analytics helps improve organizational stability and employee satisfaction by identifying at-risk individuals, evaluating turnover drivers, and adopting retention initiatives.
Advanced analytics in HR allow firms to predict and manage turnover issues. AI and data integration will improve retention analytics prediction and management skills.
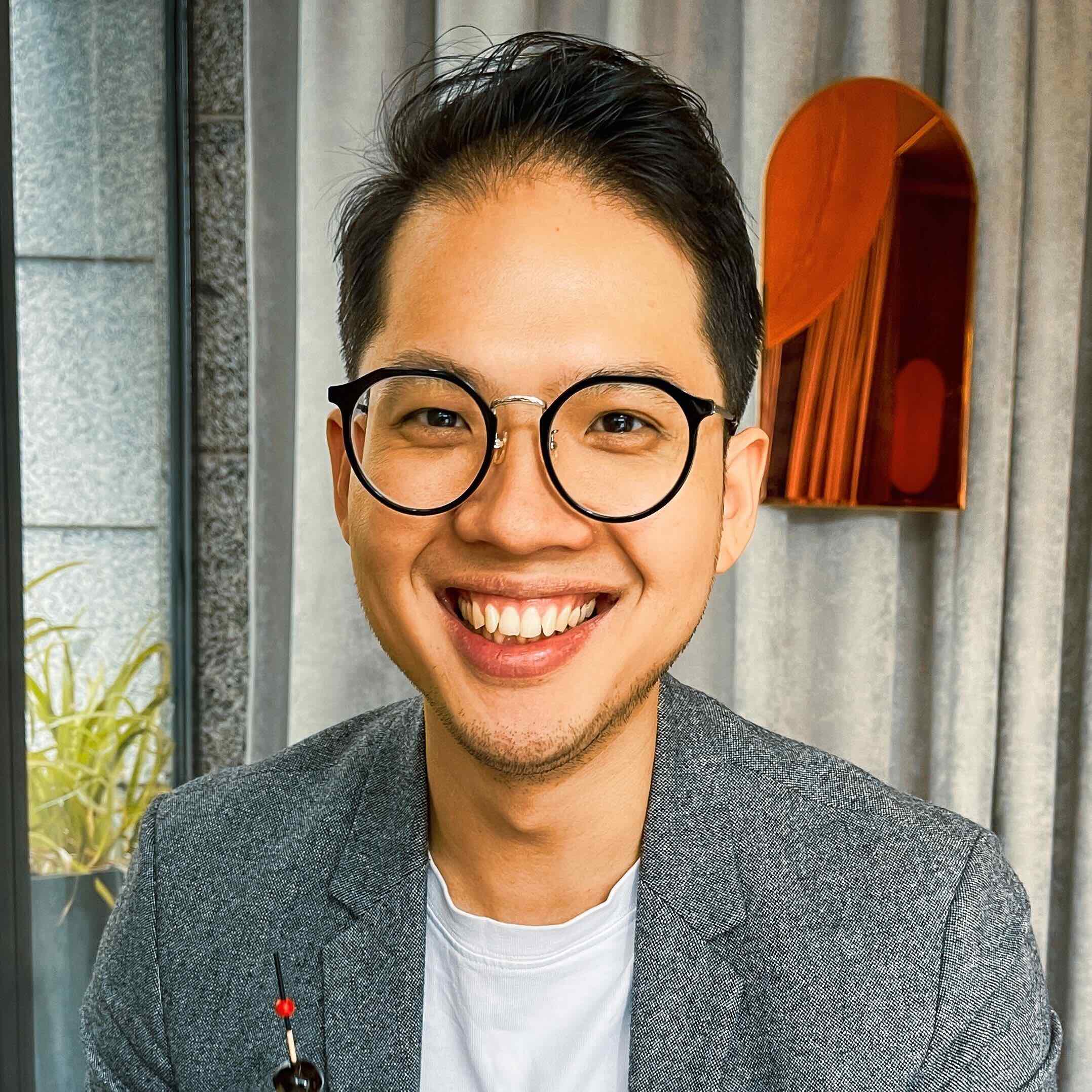
Andy is a technology & marketing leader who has delivered award-winning and world-first experiences.