Your business probably interacts with hundreds of customers across various communication channels every day. Each interaction holds a vast amount of data regarding your customer’s concerns, expectations, and even about how satisfied they are with your service.
Interaction analytics (IA) helps you analyze all this unstructured data to personalize service and avoid negative interactions.
In this article, we’ll explore what interaction analytics is and how it can benefit your company. We’ll then highlight seven software solutions you need to analyze customer interactions and improve service.
Finally, we’ll look at what the future of the IA industry looks like and how it can further aid customer experience.
This article includes:
(Click on the links to jump to a specific section)
- What is Interaction Analytics?
- Key Benefits of Interaction Analytics
- 7 Common Interaction Analytics Tools
- The Future of Interaction Analytics
Let’s get started.
What is interaction analytics?
Interaction Analytics (IA) involves using software solutions to take raw data from:
- Customer journeys.
- Customer service experiences.
- Social media interactions.
- Call recordings.
- Chat transcripts, to name a few.
The software then filters, searches, and analyzes this raw, unstructured data to turn it into structured data that provides actionable insights.
As part of the Quality Assurance (QA) process, these interaction insights can help you identify areas for improvement and offer a better customer experience.
How does it work?
The software starts by accumulating all conversations and their attached metadata, including the agent who handled the interaction, the customer’s name, and the date and time.
In the case of voice-based interaction, the data goes through a speech-to-text process to transcribe the conversation.
The software simultaneously runs a text and sentiment analysis on the customer’s and agent’s voices for signs of agitation, periods of silence, etc.
If it’s a text-based conversation, the software runs the data through text and sentiment analysis. After this step, the transcripts are sorted by category using Natural Language Processing (NLP).
What is NLP?
NLP, a form of conversational AI, allows applications to extract intent from human conversations. It then generates appropriate responses for a smoother conversational flow.
The interaction analytics solution also analyzes these interactions for specific language patterns unique to each customer. It then tags customer profiles with these characteristics for future interactions.
All this analysis provides a valuable insight into the customer’s state of mind and helps enterprises improve customer experience.
Key benefits of interaction analytics
Embracing the full power of customer interaction analytics can help your call centers and contact centers:
- Outline the customer journey to identify why the customer is reaching out for support.
- Highlight customers who need immediate attention.
- Identify key phrases and topics in customer conversations.
- Discover the root causes of customer dissatisfaction and churn.
- Identify customer behavior trends.
- Predict call volume during peak periods.
- Ensure agents stick to the script and adhere to company policies.
- Evaluate agent performance based on pre-defined KPIs (Key Performance Indicators).
- Record each agent’s knowledge base and address potential gaps through targeted coaching.
- Get real-time insights into customer sentiment to change service strategy.
- Pinpoint common customer questions to create self-service answers through chatbots, FAQs, etc.
- Train agents to solve time-consuming queries efficiently, reducing the Average Handle Time (AHT) and improving customer retention.
- Ensure your call or contact center agents adhere to regulatory compliance requirements.
- Examine conversations for specific keywords and phrases that may indicate potential fraud.
Other than these general benefits, you can also expect the following department-specific benefits.
1. Sales and marketing
Your sales and marketing division can also benefit from AI-derived customer interaction data.
Common keywords can give a hint about what they’re expecting, allowing your marketing team to tweak their strategies.
You can also measure your sales team’s effectiveness by analyzing the customer sentiment during those interactions. If a salesperson receives positive reviews, you can then use their conversation recordings to train others.
Finally, you can keep track of your brand’s social media mentions and the sentiment surrounding it to gain valuable customer insight.
2. Back-office operations
While your back-office employees may not always face customers, they are the backbone of your company.
Some of the most important business processes take place here, namely:
- Account creation and maintenance.
- Order processing.
- Payment and billing.
All these processes are often directly impacted by insights received from the contact center. So, your back office also falls under the customer experience umbrella.
Introducing analytics software to your back office can help you gain a better understanding of your business operations.
For instance, automating routine processes like data entry can help save time and increase employee satisfaction.
Similarly, you can adopt employee productivity management tools like Time Doctor to note how back-office employees utilize their time. The tool can also empower employees by providing them access to their time management data, helping them identify areas for improvement.
3. Digital experience management
Digital customer experience is entirely data-driven and dependent on analytics.
These analytics can help determine your customers’ preferred communication channels.
For instance, Millennials and Gen Z overwhelmingly prefer non-voice service, either agent-assisted or self-service. So, you may need to expand your social media teams to reach out to this growing demographic.
Similarly, customers expect faster response times on social media.
Using IA, you can measure how long they have to wait at different touchpoints and how it affects the overall Customer Satisfaction (CSAT) scores.
4. Product management
Customer feedback is essential to product/service management.
You can use call transcriptions to extract the commonly mentioned features of your product/service and the sentiment surrounding it.
You can also use IA software to keep an eye on your brand’s social media mentions on YouTube, Facebook, Twitter, and Instagram. As a result, you may be able to identify dedicated and trusted review accounts.
Using this information, you can send your product to these reviewers as a beta test – selected end-users testing the product/service to catch bugs or issues before a general release.
Since these influencers have massive younger audiences, they can help your enterprise tap into this emerging market.
Let’s explore some common IA tools you can use to reap all the above benefits.
7 common interaction analytics tools
Omnichannel contact centers receive customer information from various data sources.
The following eight tools can collect all this information into one unified dashboard, help you gain actionable customer insights, and improve agent productivity.
1. Phonetics analytics
A phonetics-based tool looks for specific sounds or strings of sounds and matches them to words and phrases in the index file.
Essentially, it correlates and converts audio data into existing words and phrases.
You can customize this tool to include different languages, dialects, regional accents, jargon, and vernacular. Similarly, you can easily add new words, like your company’s name, service tool, etc., to your existing dictionary.
However, phonetic tools can struggle with similar-sounding words. For instance, a customer describing your service as “Grade A” might get interpreted as “gray day.”
This is where conversational intelligence tools like NLP software step in to provide the necessary context.
If a customer mentions the word “website,” NLP determines whether the customer is referring to your company site or a competitor’s.
2. Speech-to-text analytics
Also known as Large Vocabulary Continuous Speech Recognition (LVCSR), this tool retains the entire conversation content rather than just specific keywords and phrases.
It gives you a full transcript of every voice interaction, allowing for root-cause analysis and a deeper insight into customer issues.
LVCSR is ideal for multichannel contact centers as the software allows for easy integration with text-based communication methods while also interpreting voice calls.
3. Text analytics
Today, most customer interactions happen on text-based channels, such as chatbots, emails, social media apps, message boards, customer surveys, etc.
A text mining solution takes all this unstructured data and evaluates it for key terms. Then, text analytics derive quantitative insights from this structured data.
For instance, the software can analyze reviews and social media mentions for price-related terms such as “expensive,” “affordable,” etc. It then notes the frequency of these words so you can decide whether to lower the price, offer a sale, or keep it the same.
These analytics can also help agents communicate with customers through real-time insights into shifting customer sentiments.
In such situations, the software often provides agents suggested responses with high success rates – for conflict resolution or even up-selling. These suggestions are especially useful for newer, less-experienced agents or when experienced agents are dealing with a demanding customer.
The software can also alert supervisors and managers of an escalating situation so they can step in if needed.
4. Sentiment analysis
AI-powered sentiment analysis provides a positive, negative, or neutral sentiment score to every customer interaction. These scores are calculated based on the number of positive and negative statements in each interaction.
Sentiment analysis can provide an excellent framework for agent training and performance reviews.
Positive interactions help agents understand which keywords and phrases customers respond to, while negative interactions help them understand which ones to avoid.
Negative interactions can also help your agents decide whether to follow up with a customer.
5. Voice of the customer (VoC) analysis
Speech, text, or sentiment analysis in isolation may not give you a complete picture of your customer’s mindsets. That’s where VoC tools help you.
They collect customer feedback across different channels using the combined power of text and speech analytics.
All this data is then analyzed to digitally track the progress of each customer interaction and identify the root causes of common issues.
For instance, you can use VoC programs to capture specific keywords on surveys. They also provide insight into customer problems, complaints, and preferences.
As a result, quality managers can implement practical changes per customer requests and improve the overall customer experience across various metrics.
VoC can also help optimize omnichannel operations, improve agent productivity, and increase customer loyalty.
6. Desktop analytics
Desktop or screen analytics provide your company with valuable information regarding your agent’s activities during a customer conversation.
Most screen monitoring tools allow you to record the agent’s desktop activity for quality monitoring purposes.
The recordings can help you evaluate their performance later. Similarly, they can even identify systems and processes leading to workflow delays.
Desktop recordings can confirm whether the agent is following the required guidelines to help customers.
7. Customer journey analysis
Customer journeys today aren’t simply from Point A to Point B.
A customer may start by browsing your website after viewing a social media ad. After purchase, they may initially contact your customer service through email or social media, finally reaching a voice call if it’s a complicated query.
The customer journey analytics solution incorporates all these different touchpoints and interactions.
Making sure all these channels work together efficiently and collectively can help reduce customer effort, leading to increased engagement.
It can also significantly improve your First Contact Resolution (FCR) metric.
The future of interaction analytics
As data collection and interpretation becomes easier, the obvious question is, what next?
These days, an interaction analytics platform integrates Artificial Intelligence (AI) and Machine Learning (ML) to form the next generation of analytics.
These will help you predict future customer behavior (predictive analytics) and how you should respond to it (prescriptive analytics).
1. Artificial intelligence (AI)
According to one estimate, about 90% of all digital data is unstructured. When used right, this data can be highly beneficial for businesses.
All the tools mentioned above use AI to recognize patterns and suggest relevant actions by analyzing this unstructured customer data.
Today, most contact centers utilize AI-based automation to:
- Collect customer experience ratings.
- Conduct customer surveys.
- Offer 24/7 omnichannel support.
AI can also help analyze past behavior to predict future buying behavior, allowing you to offer hyper-personalized customer service.
2. Machine learning (ML)
Confused about the difference between AI and ML?
Machine learning (ML) is a subset of AI, allowing machines to learn from existing data without any direct instruction. On the other hand, AI aims to mimic human cognitive capabilities such as problem-solving and decision-making.
Based on this definition, ML helps contact centers by observing manually-scored customer interactions. It then learns these scoring criteria, letting you automate the process.
The more it scores, the more it learns and the better it becomes.
In this way, it helps AI become more efficient and go beyond its programming to deliver a higher level of customer service.
3. Predictive and prescriptive analytics
Predictive analytics uses past data diagnostics to predict what’s most likely to happen, while prescriptive analytics recommend the best course of action.
Augmented analytics then come into play and help you map out your next move.
What is augmented analytics?
These analytics use tools such as AI and ML to expand a human’s ability to interact with the existing data contextually.
For instance, instead of just providing information that sales in a specific county are down 10% from the previous quarter, augmented analytics helps you understand why they’re down and how to remedy it.
As a result, you can expect to:
- Save on operational costs.
- Speed up decision-making.
- Increase employee trust regarding automation tools.
Augmented analytics can also provide better insight into your customer base and their pain points, allowing you to provide proactive and more targeted service.
Doing this will reduce customer effort at each step of the process, increasing engagement and loyalty.
No wonder Gartner named it as one of the most promising data and analytics technology trends!
Wrapping up
Interaction analytics provide a complete picture of the customer’s expectations, history with your brand, preferences, and much more. As a result, you can personalize your services, leading to increased customer engagement and retention.
IA tools can also help increase contact center productivity by optimizing workflows and automating routine processes.
By identifying emerging customer trends and adopting disruptive technologies, you can offer a higher level of customer service and increase your profit margins.
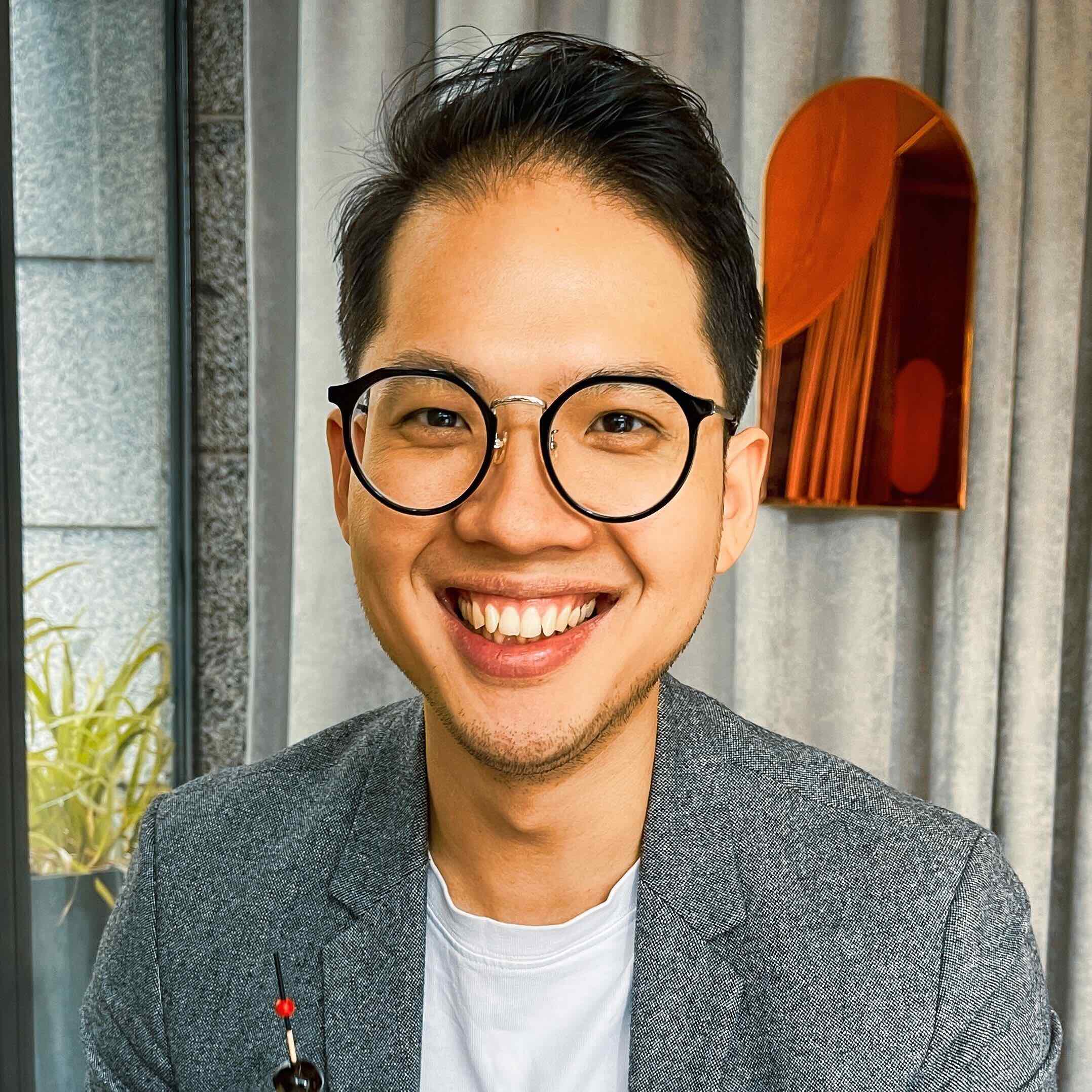
Andy is a technology & marketing leader who has delivered award-winning and world-first experiences.